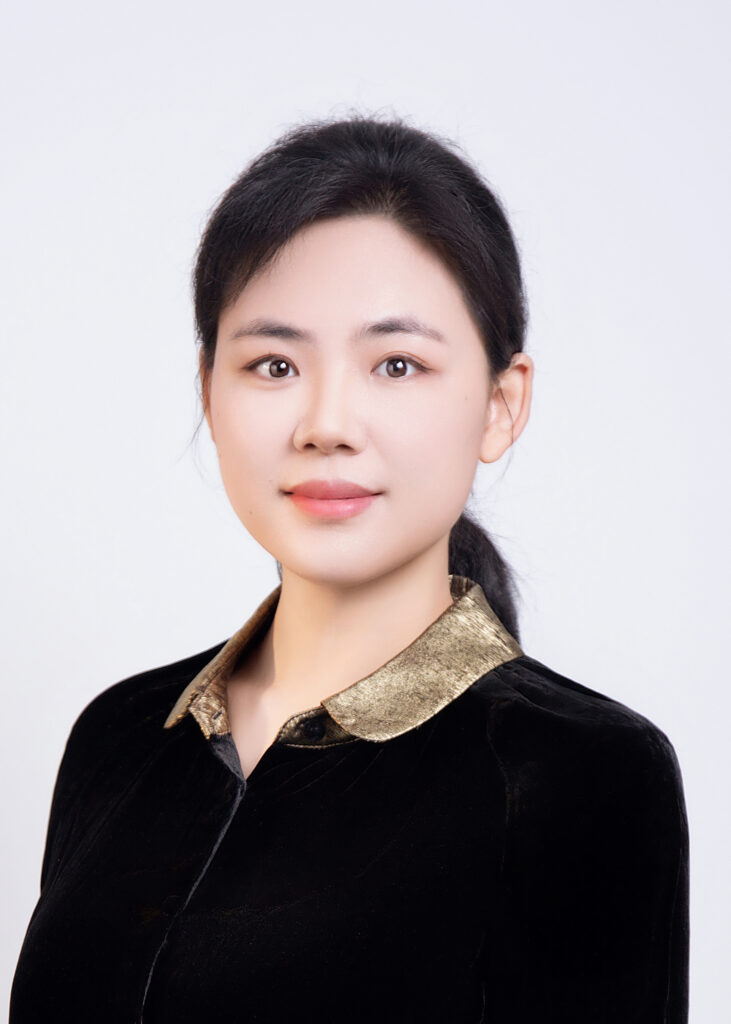
Jing Liu
National Key Laboratory of Radar Signal Processing, Xidian University, China
Prof. Jing Liu is currently at the National Laboratory of Radar Signal Processing, Xidian University. She studied abroad in The University of Western Australia during 2015-2017, and received the doctor’s degree in Control Science and Engineering from Harbin Engineering University in 2018. Her research interests are machine learning, radar multi-dimensional signal processing, array signal processing and target detection. She has published more than 20 papers, applied for more than 30 patents, and presided over 8 scientific research projects such as the National Natural Science Foundation. Related to these academic researches and their core courses, she has won: First prize in Electronic Experiment Skill Competition of young and middle-aged teachers in universities of Shaanxi province; second prize in the Young Teachers’ Teaching Competition of Xidian University, and first prize in the Young Teachers’ Teaching Competition of School of Electronic Engineering.
Training Feature Generation and Machine Learning based Radar Detection for Small Targets
Detecting a small target is a hot and challenging topic in the field of signal processing and machine learning. Especially for radar signal processing, the low signal-to-clutter ratio (SCR) of small targets results in the targets being particularly easily overwhelmed by the clutter. In this presentation, we will provide strategies based on specific machine learning integrated decision tree, small target signal repository, and fast-built real-time training feature data generation for small targets. It takes advantage of machine learning, and attains the highest probability of detecting small targets under the low SCR.
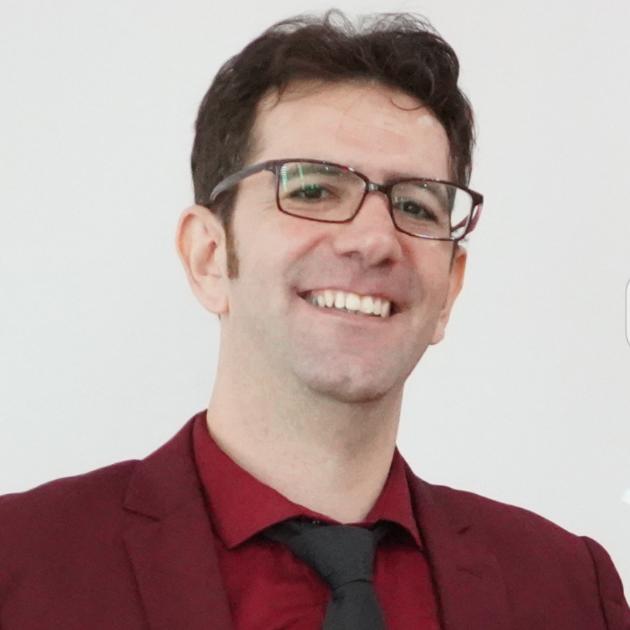
Mansour Amini
School of Languages, Literacies, and Translation, Universiti Sains Malaysia
Dr. Mansour Amini is an interdisciplinary researcher and a senior lecturer from the School of Languages, Literacies, and Translation, Universiti Sains Malaysia (USM). He has a Diploma in Mathematics and Physics, a B.A. in English Language and Literature, an M.A. in English Language Teaching, and a PhD in Translation Studies. He has published 94 articles in Translation, Education, Humanities, and Social Sciences, and is the principal investigator of several research projects. He has conducted workshops, seminars, and talks on Thesis Writing and Research Methodology in Malaysia, Thailand, Indonesia, Iran, and China. Dr. Mansour Amini is a reviewer, editing manager, and editorial board member of several academic journals. He has tried to link research with teaching practice by applying the lessons learned from authentic research outputs to teaching scenarios.
AI in Translation Studies: Current Trends, Challenges, and Prospects
This paper presents a comprehensive examination of the integration of artificial intelligence (AI) in translation studies. It delves into a variety of AI methodologies employed in translation, including statistical machine translation, rule-based machine translation, neural machine translation, and hybrid machine translation. Each model’s advantages, limitations, and specific applications within translation studies are thoroughly analyzed. In addition to model-specific evaluations, the paper reviews diverse techniques for assessing the effectiveness of AI models in translation, highlighting their respective strengths and weaknesses. The study addresses significant challenges inherent in AI translation, such as the accurate handling of idioms, metaphors, and cultural nuances. Furthermore, it explores potential research trajectories aimed at enhancing AI-driven translation. The review extends beyond technical aspects, discussing AI’s portrayal in literature and the arts, examining academic opportunities, and investigating the broader impacts on human lives. Ethical and social considerations, such as job displacement, data privacy, and issues of bias and fairness, are scrutinized to provide a holistic view of AI in translation. The paper concludes by summarizing key findings, implications, and offering recommendations for future research directions. This review provides valuable insights into the current state of AI in translation, highlighting its transformative potential for the field and laying the groundwork for future advancements in AI-based translation studies.
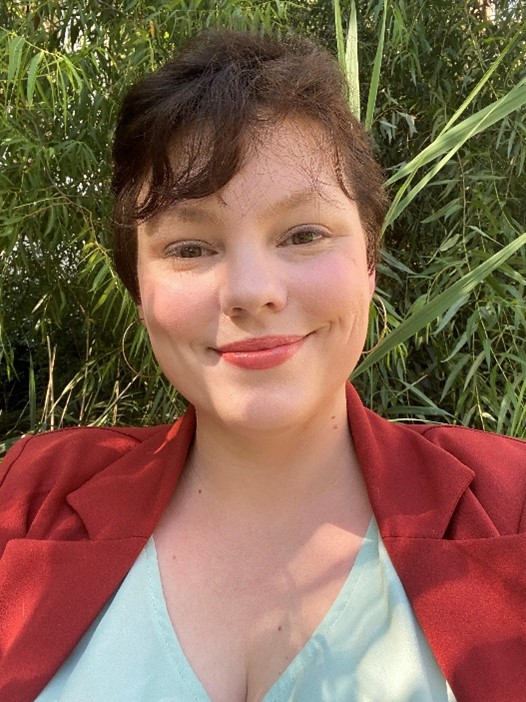
Rita Detrick
Department of STEM Education and Professional Studies, Old Dominion University, USA
Rita Detrick is currently a Ph.D. student in the Educational Psychology and Program Evaluation program at Old Dominion University. She obtained her bachelor’s degree in psychology from Troy University, followed by a master’s degree in social science. Professionally, she works as an Innovation Strategist, leading and contributing to education, credentialing, and workforce solutions. Her research interests include artificial intelligence in education, technology-enhanced learning, and addressing the challenges faced by nontraditional students. Rita has contributed to numerous papers and various research projects and played a key role in developing innovative strategies in her field.
Academic Writing and the Impact of Student-Generative AI Interactions
Although Generative AI (GenAI) presents transformative opportunities for academic writing, knowledge of student-GenAI interaction (SAI) is still emerging. Further research is needed to develop an understanding of the interaction patterns that students display while working with AI on an academic writing task and how those various patterns relate to task performance. This study examined how 36 Chinese graduate students interacted with a GenAI writing system to complete an academic writing task. Students were grouped by their AI literacy level. To support the SAI identification process, three data sources produced during the students’ writing activity with the GenAI system were analyzed. These included think-aloud protocols, screen recordings, and chat histories. Epistemic network analysis (ENA) was used to reveal SAI patterns across different levels of AI literacy. High AI literacy students were found to be more collaborative and accepting of GenAI’s suggestions, while low AI literacy students worked more independently. The Wilcoxon rank-sum (Mann-Whitney U) test was leveraged to assess the performance of the two groups and revealed statistical differences across all evaluation areas (content, structure/organization, expression). This research provides insights into the design and implementation of generative AI agents for writing tasks and the teaching methods involving AI assistance.